Researchers have developed a new memristive device capable of self-compliance, multilevel operation, and a crossbar array for forming neural networks.
Neuromorphic or brain-like computing systems offer several advantages for artificial intelligence applications. Resistive random-access memory (RRAM), a type of emerging semiconductor technology, exhibits potential for neuromorphic computing applications. However, conventional designs suffer from overshoot currents, leading to reliability issues and requiring external current compliance settings. Now, researchers have developed a new memristive device that achieves self-compliance, enabling crossbar array and multilevel operation, paving the way toward high-performance neuromorphic computing.
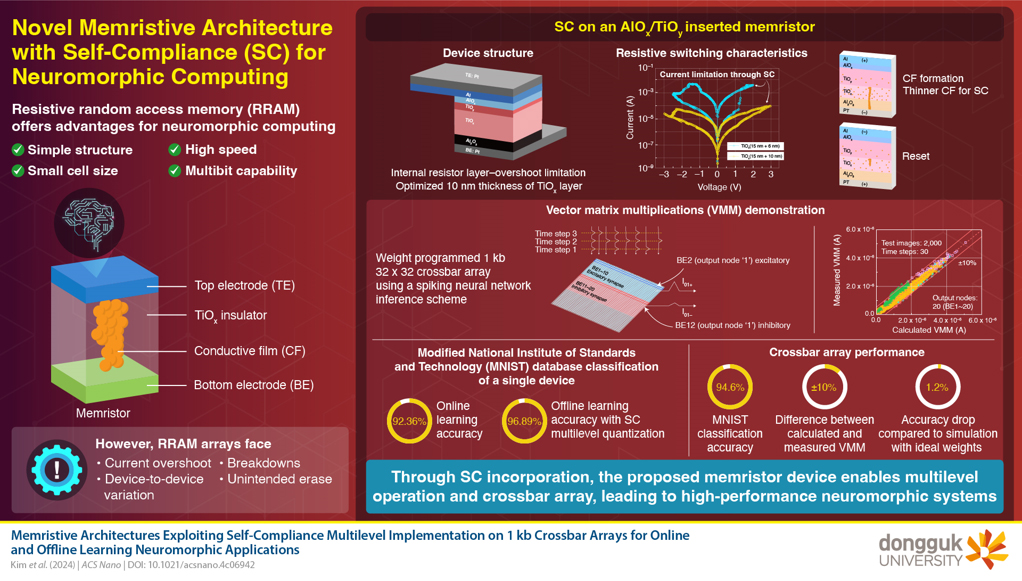
<Researchers from Dongguk University propose a memristor device and crossbar array>
In the recent years, artificial intelligence (AI) and Internet of Things have progressed rapidly, driving advancements in areas like speech recognition, image classification in autonomous vehicles, and large language models like ChatGPT. A key element in AI is deep learning, which requires parallel processing of large amounts of data—an area where traditional computers still struggle with efficiency. Neuromorphic or brain-like computing systems, consisting of artificial neurons and synapses, offer low power consumption and efficient data processing.
One of the most promising semiconductor technologies for neuromorphic computing is the resistive random-access memory (RRAM), a type of memristive device. Memristive devices have the unique ability to “remember” past electrical states. In RRAM, this memory effect arises from the formation and dissolution of a conductive filament (CF) in the insulator layer of its metal-insulator-metal structure. Metal oxide insulators play an essential role in this process. However, while titanium-oxide-based RRAMs offer several advantages, they suffer from device-to-device variations, caused by overshoot currents during CF formation. This can lead to breakdowns or unintended memory erasure. Current methods to mitigate overshoot currents require the addition of transistors or external current compliance (CC) settings, increasing complexity.
In a breakthrough now, a research team from South Korea, led by Professor Sungjun Kim from the Division of Electronics and Electrical Engineering at Dongguk University, developed a self-compliance (SC) memristor device that overcomes these issues. Elaborating further, Prof. Kim says, “In this study, we achieved SC on a high-density two-terminal memristor and implemented vector-matrix multiplication (VMM), the core of AI semiconductor computation, on a 32 x 32 memristor array.” Their study was made
available online on August 21, 2024, and published in Volume 18, Issue 36 of ACS Nano on September 10, 2024.
The innovative memristor device has an aluminum oxide/titanium-oxide (AlOx/TiOy) layer on top of the insulator layer. This layer acts as an internal resistor, preventing overshoot currents by controlling the thickness of the CF formed during switching, which achieves SC. The researchers fine-tuned the TiOy layer to 10 nanometers, improving the device's performance.
Through a series of experiments, the researchers demonstrated the device’s consistent switching characteristics without external CC and reliable multilevel operation with low power consumption. They also studied the device’s long-term potentiation (LTP) and long-term depression (LTD) characteristics, which represent the strength of synaptic connections between neurons in neuromorphic computing systems.
Using these characteristics, they simulated neural networks based on the device to classify images from the well-known MNIST database. Results revealed an online learning accuracy of 92.36%. Furthermore, offline learning neural networks that leveraged the device’s SC multilevel mode achieved an accuracy of 96.89%.
Ultimately, the researchers built a neural network using a 32 x 32 crossbar array of their memristors to demonstrate spiking neural network (SNN)-based VMM operations. SNNs, which mimic the computation processes of the brain, are known for their low power consumption. The crossbar array-based neural network achieved a 94.6% classification accuracy on the MNIST dataset, with only a 1.2% accuracy drop compared to simulation results, which shows its exceptional capabilities.
“Memristor arrays will be pivotal in next-generation computing architectures due to their speed, efficiency, and scalability,“ remarks Prof. Kim. “Beyond neuromorphic computing, they have a wide range of potential applications, including non-volatile memory, IoT, machine learning, and cryptography. Furthermore, neural processing units, specialized for AI operations, require memory chips tailored for VMM operations such as the high yield memristor array developed in this study,” he adds.
In summary, this innovative device opens avenues for the development of high-performance, energy-efficient neuromorphic computing systems, unlocking advanced new AI applications.
Reference
Title of original paper: Memristive Architectures Exploiting Self-Compliance Multilevel Implementation on 1
kb Crossbar Arrays for Online and Offline
Learning Neuromorphic Applications
Journal: ACS Nano
Additional information for EurekAlert
Latest Article Publishing Date:
September 10, 2024
Method of Research: Experimental Study
Subject of Research: Not applicable
COI Statement: The authors declare no competing financial interest.
About the institute
Dongguk University, founded in 1906, is located in Seoul, South Korea. It comprises 13 colleges that cover a variety of disciplines and has local campuses in Gyeongju, Goyang, and Los Angeles. The university has 1300 professors who conduct independent research and 18,000 students undertaking studies in a variety of disciplines. Interaction between disciplines is one of the strengths on which Dongguk prides itself; the university encourages researchers to work across disciplines in Information Technology, Bio Technology, CT, and Buddhism.
About the author
Sungjun Kim is a Professor at Dongguk University, Seoul, Republic of Korea. Professor Kim received his Ph.D. in Electrical Engineering from Seoul National University, Seoul, Republic of Korea, in 2017. From 2017 to 2018, he was a Senior Engineer at Samsung Electronics Company Ltd., Republic of Korea. He joined Chungbuk National University, Republic of Korea, as an Assistant Professor in 2018. His research topics mainly include memristors, ferroelectric memories, other emerging memories, and neuromorphic semiconductors. He has published more than 280 publications in peer-reviewed journals with an h-index of 33 (Scopus, August 2024).